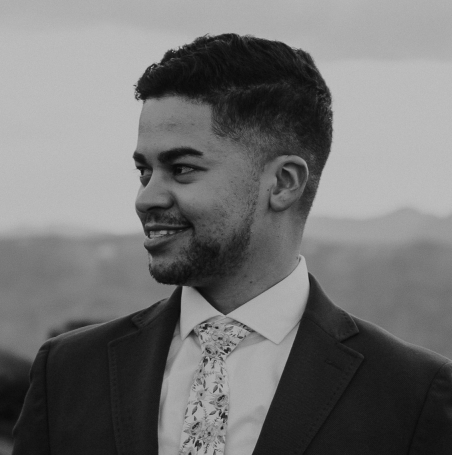
I am a machine learning research scientist at the National Renewable Energy Lab (NREL) with broad expertise in deep learning and foundation models. I lead a US Dept. of Energy ASCR-funded AI for Science project where we are researching hallucination mitigation, probabilistic reasoning, and multimodality in conversational Assistants. Our vision is to build Assistants that aid scientists by accelerating computational experiment-driven discovery. At NREL, I have applied my expertise in areas including building energy management and protein engineering. My contributions at NREL have been recognized with an Outstanding Mentor Award (2023) and a Postdoctoral Publication Award (2024).
[CV][Google scholar] [Github]
Contact: Patrick[dot]Emami[at]nrel[dot][gov]
News
- February 2025 Saumya’s paper “On the effectiveness of neural operators at zero-shot weather downscaling” has been accepted for publication in the Environmental Data Science journal!
- January 2025 Our paper “SysCaps: Language Interfaces for Simulation Surrogates of Complex Systems” is accepted as a poster at ICLR 2025!
- December 2024 I am serving as a Co-Organizer and Mentorship Chair for the ICLR’25 Workshop on Tackling Climate Change with Machine Learning!
- December 2024 Our survey paper “Deep generative models in energy system applications: Review, challenges, and future directions” has been published in Applied Energy
- October 2024 My proposal “Theseus: A Computational Science Foundation Model” was awarded by DOE/ASCR ($2.35M/3 years)
Recent Papers
SysCaps: Language Interfaces for Simulation Surrogates of Complex Systems
Augmenting surrogate models for complex systems with natural language interfaces.
Non-Stationary Policy Learning for Multi-Timescale Multi-Agent Reinforcement Learning
Framework for learning non-stationary policies in multi-timescale MARL by leveraging periodicity.
BuildingsBench: A Large-Scale Dataset of 900K Buildings and Benchmark for Short-Term Load Forecasting
Platform for studying large-scale pretraining + zero-shot generalization/finetuning of building load forecasting models.
Plug & Play Directed Evolution of Proteins with Gradient-based Discrete MCMC
Also presented at NeurIPS’22 Workshop on Machine Learning in Structural Biology
A fast MCMC sampler for discovering variants by mixing and matching unsupervised evolutionary sequence models with supervised models that map sequence to protein function.
See my CV for a complete list including older publications.
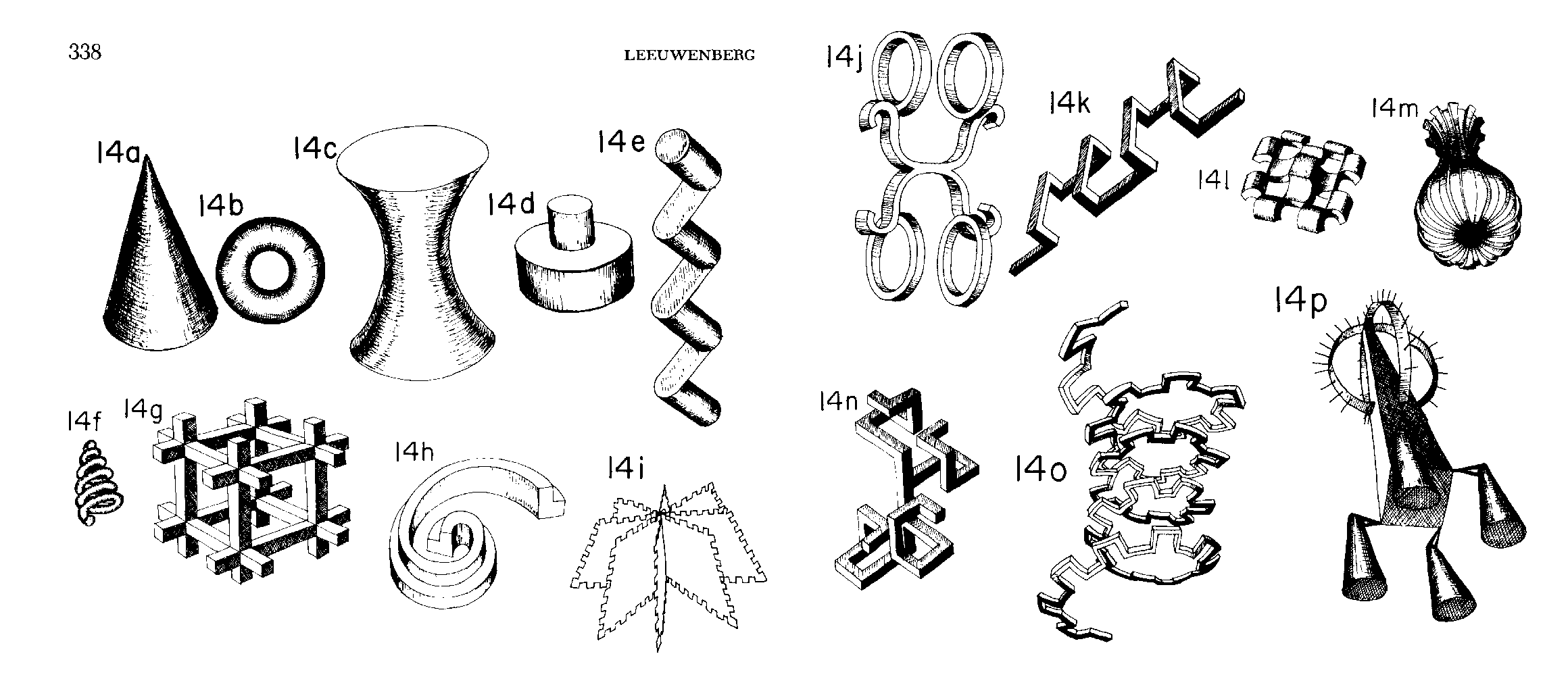
Leeuwenberg, E. L. J. "A Perceptual Coding Language for Visual and Auditory Patterns." The American Journal of Psychology, vol. 84, no. 3, 1971, pg. 338.